In a time where decisions are based on data, healthcare stands at the precipice of a transformative journey propelled by predictive analytics. Picture this: a world where ailments are foreseen and prevented, resources are allocated with precision, and patient care is tailored to individual needs. Welcome to the future of healthcare, where predictive analytics reigns supreme.
The global healthcare predictive analytics market is expected to grow at a compound annual growth rate (CAGR) of 23.2% from 2021 to 2028, reaching $19.5 billion, according to a Grand View Research analysis. However, what precisely is predictive analytics in the medical field, and how does it operate? Let’s explore the wonders and complexities of this state-of-the-art technology.
What is Predictive Analytics in Healthcare?
Predictive analytics employs data analysis, statistical algorithms, and machine learning to forecast future outcomes using historical and real-time data. This transformative technology empowers healthcare providers to anticipate health risks, optimize treatments, and improve operations.
How Predictive Analytics Works in Healthcare Settings?
At its core, predictive analytics utilizes advanced algorithms to analyze vast datasets comprising patient demographics, clinical history, diagnostic tests, and treatment outcomes. By identifying patterns, correlations, and anomalies within these data streams, predictive models can generate actionable insights to guide clinical decision-making and resource allocation.
Healthcare organizations use predictive analytics in many areas, from forecasting disease outbreaks and improving medication compliance to pinpointing patients at high risk for early intervention. As these algorithms are continuously refined and validated, they evolve to accommodate changing healthcare needs and emerging patient priorities.
Use of Predictive Analytics in Healthcare
Identifying Patterns and Trends in Patient Data
Healthcare organizations can uncover crucial correlations and associations for informed decision-making by using predictive analytics to mine disparate data sources. This data ranges from demographics to clinical parameters and treatment outcomes. Sophisticated algorithms and machine learning techniques empower these organizations to identify subtle patterns and trends in vast troves of patient data. Such insights would otherwise elude human observation. In this way, predictive analytics enables clinicians and administrators to unlock the hidden value in patient data.
Using predictive analytics, healthcare providers can proactively pinpoint individuals prone to chronic conditions like diabetes or heart disease, based on lifestyle, genetics, and environment. By grouping patients by risk profile, healthcare teams can efficiently allocate resources, prioritize preventive care, and create personalized plans to mitigate health risks.
Risk Assessment and Stratification
One of the key uses of predictive analytics in healthcare is assessing and categorizing risk. By examining past patient information and clinical markers, predictive models can accurately foresee the chance of negative outcomes like hospital readmissions, patients not taking medication properly, or worsening of illness. With this knowledge, healthcare providers can take preventive action, implement focused interventions, and empower patients in proactive self-care approaches.
Using predictive analytics to identify high-risk patients, healthcare organizations reduced hospital readmissions and improved outcomes for heart failure patients, according to research in the Journal of the American Medical Informatics Association. By deploying targeted interventions for patients predicted to be at risk of readmission, they achieved significant reductions in readmission rates, leading to better patient outcomes and cost savings.
Treatment Optimization and Personalized Medicine
Predictive analytics is essential for optimizing treatment plans and providing patients with individualized care in the era of precision medicine. Predictive models, which examine patient-specific data such as therapy response profiles, genetic markers, and biomarkers, can help doctors choose the best treatments based on each patient’s unique needs.
Studies have shown that predictive analytics can enhance medication adherence and treatment compliance among patients with chronic conditions, such as diabetes and hypertension. By leveraging predictive algorithms to predict medication adherence patterns and identify at-risk individuals, healthcare providers can intervene proactively, offer targeted support, and improve patient adherence rates, thereby optimizing treatment outcomes and reducing healthcare costs.
Resource Allocation and Operational Efficiency
Predictive analytics offers immense potential to optimize resource allocation and enhance operational efficiency in healthcare organizations. By forecasting patient demand, predicting resource use patterns, and optimizing workflow, predictive models allow healthcare administrators to allocate personnel, equipment, and facilities more efficiently. This ensures optimal resource utilization and minimizes bottlenecks.
The influence of predictive analytics on operational efficiency within healthcare organizations is highlighted by research undertaken by the Healthcare Information and Management Systems Society (HIMSS). Healthcare institutions can optimize bed capacity, reduce wait times, and streamline operations by utilizing predictive models to forecast patient flow and anticipate personnel needs.
Benefits of Predictive Analytics in Healthcare
Predictive analytics presents a dynamic and transformational force in the rapidly evolving field of modern healthcare, with numerous advantages that go well beyond established healthcare paradigms. Let’s explore the many benefits of predictive analytics in transforming clinical decision-making, patient care, and the provision of healthcare.
Early Disease Detection and Prevention
The potential of predictive analytics to support early disease detection and prevention in the healthcare industry is among its most persuasive advantages. Predictive models assess patient data, such as demographics, medical history, and lifestyle factors, to determine who is more likely to develop a particular disease or experience health issues. By taking a proactive approach, healthcare providers can improve long-term results and prevent possible health crises by empowering patients to adopt healthier lifestyles, implementing preventive measures, and intervening early.
A study published in the Journal of Medical Internet Research demonstrates the effectiveness of using predictive analytics to identify individuals at high risk of developing type 2 diabetes. By applying machine learning algorithms to analyze electronic health records and clinical data, the researchers significantly improved early detection of prediabetes. This enables timely interventions and lifestyle changes to prevent progression to type 2 diabetes.
Improved Patient Outcomes and Quality of Care
There is great potential for improving patient outcomes and raising the standard of care provided in all healthcare settings with predictive analytics. Healthcare professionals can customize interventions to each patient’s preferences and characteristics by using predictive models to anticipate requirements, personalize treatment plans, and optimize care pathways. This leads to more effective therapies and better clinical outcomes.
A recent study published in JAMA shows how predictive analytics can reduce hospital-acquired infections and adverse events. Researchers used predictive algorithms to identify high-risk patients and enable healthcare teams to target them with interventions like increased monitoring and infection control. This approach significantly decreased adverse outcomes and improved patient safety.
Cost Reduction and Financial Efficiency
Predictive analytics presents a strong case for generating cost savings and improving financial efficiency inside healthcare organizations in a time of rising healthcare expenses and limited resources. Predictive models help healthcare providers achieve higher operational efficiency and cost-effectiveness while preserving high-quality patient care by streamlining workflows, optimizing resource allocation, and reducing needless interventions.
McKinsey & Company reports that predictive analytics can realize substantial healthcare cost savings by targeting high-cost patient groups, optimizing care delivery, and preventing unnecessary healthcare usage. This data-driven approach reduces expenditures across chronic disease management, hospital readmissions, emergency services, and more. Healthcare organizations leveraging predictive insights stand to achieve significant financial gains.
Enhanced Population Health Management
A key component of population health management techniques is predictive analytics, which enables healthcare companies to proactively meet the requirements of a variety of patient populations and significantly improve health outcomes. Predictive models assist healthcare professionals in mitigating inequities, optimizing resource allocation, and promoting health equity across communities by assessing population-level data, identifying susceptible subgroups, and devising customized interventions.
The influence of predictive analytics on population health management programs targeted at lowering the burden of chronic disease and enhancing the provision of preventative treatment is demonstrated by research published in Health Affairs. Healthcare companies saw notable gains in patient engagement, health outcomes, and population health indicators by using predictive algorithms to stratify people according to risk profiles and health needs.
Risks of Predictive Analytics in Healthcare
Predictive analytics adoption is not without inherent dangers and limitations, despite the enormous promise it possesses to transform healthcare delivery and enhance patient outcomes. To ensure the responsible and ethical use of predictive models in clinical practice, it is imperative that we identify and address these possible hazards as we navigate the complicated environment of healthcare analytics.
Data Privacy and Security Concerns
Data security and privacy are two of the main issues with predictive analytics in the healthcare industry. The accumulation of extensive amounts of confidential patient data by healthcare institutions, such as genetic data, medical records, and demographic profiles, raises the possibility of data breaches and unauthorized access. In addition, the incorporation of other data sources—such as consumer behavior and social determinants of health—increases privacy problems and poses moral conundrums with regard to consent and data ownership.
The difficulties in protecting patient privacy in the age of predictive analytics are brought to light by a study that was published in the Journal of the American Medical Informatics Association. Researchers stress that in order to reduce privacy threats and guarantee compliance with strict regulatory frameworks, such the Health Insurance Portability and Accountability Act (HIPAA), strong data encryption mechanisms, access controls, and anonymization techniques are essential.
Potential for Biases and Discrimination in Algorithms
Algorithmic prejudice and discrimination is a serious issue that predictive analytics in healthcare may introduce. Predictive models may unintentionally reinforce or magnify preexisting biases in healthcare systems, such as differences in treatment results, diagnosis rates, and access to care, since they rely on past data to make future predictions. Furthermore, by disproportionately affecting vulnerable communities and widening gaps in health outcomes, algorithmic biases might worsen healthcare inequities.
A study that was published in Science Translational Medicine emphasizes how algorithmic decision-making systems must be transparent and accountable in order to reduce prejudice and guarantee equity in the provision of healthcare. Healthcare organizations may improve patient-centered care and social justice by enhancing the trustworthiness and equity of predictive analytics solutions through the use of rigorous validation methods, bias detection algorithms, and model interpretability methodologies.
Accuracy and Reliability of Predictive Models
Despite advancements in machine learning and data science, predictive models in healthcare analytics still face critical uncertainties. These models provide probabilities, not certainties, so their accuracy depends on factors like data quality, sample size, and model complexity. Poor data or modeling can lead to unreliable clinical predictions.
The predictive accuracy of machine learning models in clinical settings is examined in a meta-analysis published in JAMA Internal Medicine, demonstrating the variation in performance across various disease domains and healthcare situations. In order to improve the clinical usefulness and dependability of predictive analytics tools in healthcare practice, researchers stress the significance of ongoing model validation, real-time feedback loops, and physician input.
Examples of Predictive Analytics in Healthcare
Predictive analytics is gaining momentum across healthcare, spurring innovative solutions that enhance clinical decision-making and care delivery. Real-world examples showcase its transformative potential—from forecasting disease outbreaks to predicting patient readmissions, predictive analytics optimizes resource allocation and improves patient outcomes.
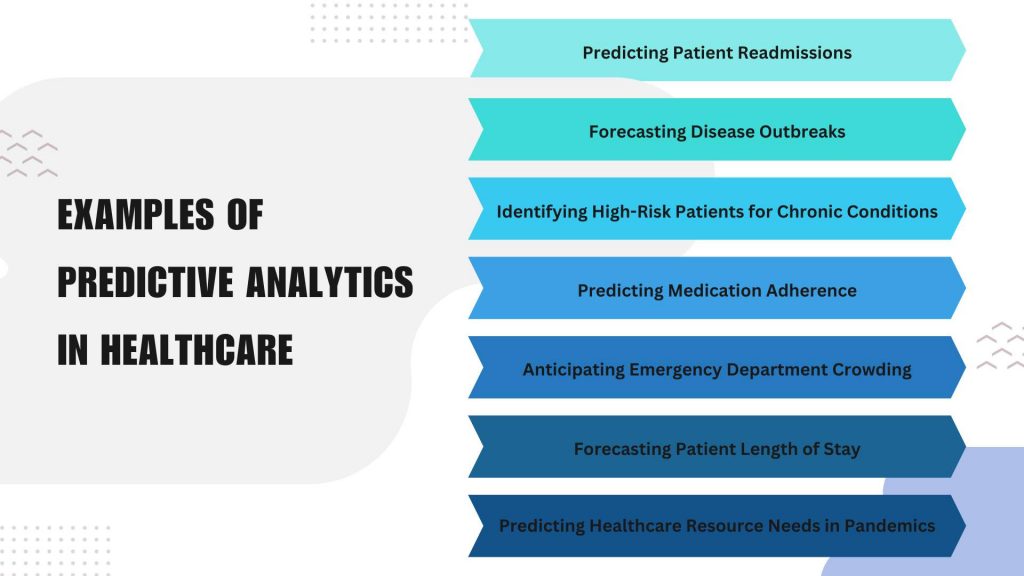
1. Predicting Patient Readmissions
Hospital readmissions are a major problem for healthcare systems around the globe because they raise expenses and lower patient satisfaction. Predictive analytics uses clinical markers, socioeconomic determinants of health, and past patient data to provide a proactive strategy to reduce readmission risk. Healthcare professionals can optimize care transitions, reduce unnecessary hospitalizations, and adjust post-discharge interventions by identifying individuals who are at heightened risk of readmission.
A study that was published in the Journal of General Internal Medicine shows how effective predictive analytics is at lowering readmission rates for patients with heart failure. Healthcare organizations experienced notable decreases in readmission rates and better patient outcomes by using predictive models into care coordination initiatives and remote monitoring programs. This improved patient outcomes led to higher quality treatment and lower healthcare expenses.
2. Forecasting Disease Outbreaks
Public health agencies use predictive analytics to foresee and control the spread of infectious diseases like influenza, Zika virus, and COVID-19. Analyzing epidemiological data, environmental factors, and population movement patterns, predictive models allow for early detection of disease outbreaks, swift resource allocation, and targeted interventions to limit transmission.
Predictive analytics was essential in predicting the COVID-19 pandemic’s progress, directing public health response initiatives, and influencing policy choices. Epidemiologists produced precise estimates of COVID-19 transmission dynamics by combining information from several sources, including as genetic sequencing, mobility data, and healthcare use rates. This allowed for the implementation of preventative measures like lockdowns, testing programs, and vaccination campaigns.
3. Identifying High-Risk Patients for Chronic Conditions
Chronic conditions that require long-term care and have concomitant conditions, such diabetes, hypertension, and asthma, provide serious healthcare issues. Through the examination of clinical data, lifestyle factors, and genetic predispositions, predictive analytics enables the early identification of patients who are at a high risk of developing chronic illnesses. Healthcare professionals can carry out preventative interventions, encourage self-management techniques, and enhance long-term health outcomes by grouping patients according to their risk profiles.
Studies that have been published in Diabetes Care show how predictive analytics can be used to identify those who are at risk of type 2 diabetes and to apply specific lifestyle treatments. Healthcare teams reduced the incidence rates of diabetes and improved population health metrics by using predictive models to evaluate risk variables like obesity, family history, and glucose levels. These results demonstrate the importance of proactive disease preventive efforts.
4. Predicting Medication Adherence
A major problem in healthcare is medication non-adherence, which raises hospitalization rates, treatment costs, and less-than-ideal treatment results. A data-driven method for evaluating patient adherence patterns, identifying those who are at risk, and taking proactive measures to increase prescription adherence rates is provided by predictive analytics.
Predictive analytics has an effect on patients with chronic illnesses’ adherence to medicine, as demonstrated by a study published in JAMA Internal Medicine. Researchers created predictive models to identify patients at risk of non-adherence and customized interventions, like medication reminders, patient education, and telehealth support, improving adherence rates and clinical outcomes. These models were developed by analyzing pharmacy and electronic health record (EHR) data.
5. Anticipating Emergency Department Crowding
Overcrowding in emergency departments (EDs) presents serious problems for healthcare systems, as it jeopardizes patient safety, lengthens wait times, and puts a burden on scarce resources. Healthcare organizations can use predictive analytics to forecast patterns of ED consumption, foresee spikes in demand, and take proactive steps to optimize patient flow and resource allocation.
Reducing ED crowding and increasing patient throughput are two benefits of predictive analytics, as demonstrated by a study published in Health Affairs. Healthcare facilities implemented capacity management strategies, including real-time monitoring, staff allocation, and diversion protocols, by using predictive models to forecast patient arrivals, acuity levels, and resource needs. This led to decreased wait times, improved patient satisfaction, and improved clinical outcomes.
6. Forecasting Patient Length of Stay
By projecting patient length of stay (LOS) and bed occupancy rates, predictive analytics is essential to streamlining hospital operations and allocating resources. Predictive models help healthcare providers anticipate length of stay (LOS), maximize bed utilization, and expedite discharge procedures to lower hospital overcrowding and improve operational efficiency. They do this by evaluating clinical data, severity scores, and discharge planning measures.
The effect of predictive analytics on resource allocation and LOS forecast accuracy in hospital settings is investigated in a study that was published in the Journal of Hospital Medicine. Healthcare companies saw notable gains in LOS estimation accuracy, bed turnover rates, and patient flow dynamics through the integration of predictive models into electronic health record (EHR) systems and care coordination processes. This led to increased throughput and cost savings.
7. Predicting Healthcare Resource Needs in Pandemics
Predictive analytics is essential for predicting the demand for healthcare resources, such as hospital beds, ventilators, and personal protective equipment (PPE), during public health emergencies like pandemics and natural disasters. Predictive models help healthcare organizations foresee spikes in demand, strategically allocate resources, and effectively respond to emergencies by evaluating epidemiological data, disease transmission dynamics, and population health indices.
It is crucial for pandemic preparedness and response, as the COVID-19 pandemic demonstrated. Public health authorities and healthcare systems were better equipped to handle the changing challenges brought about by the pandemic by using predictive models to project healthcare resource needs, identify high-risk populations, and optimize vaccination distribution strategies.
Predictive analytics has the potential to revolutionize the healthcare industry at every stage, from emergency response and pandemic preparedness to chronic illness management and preventive treatment. This is made clear when we examine these many examples of its uses in the field.
Future of Predictive Analytics in Healthcare
We stand at the dawn of a new era of healthcare innovation, in which predictive analytics holds tremendous potential to transform how we deliver and experience healthcare. From integration with wearable technology to advancements in artificial intelligence, exciting trends in predictive analytics promise to revolutionize the healthcare landscape.
Integration with Wearable Technology and IoT Devices
Predictive analytics in healthcare has never had more chances thanks to the widespread use of wearable technology, including smartwatches, fitness trackers, and sensors for remote monitoring. Predictive models are able to instantly deliver real-time insights into patient health status, activity levels, and vital signs by seamlessly combining data streams from wearable devices with clinical databases and electronic health records (EHRs). Proactive health monitoring, early identification of health anomalies, and individualized interventions catered to the specific needs of each patient are made possible by this constant flow of data.
A report by Allied Market Research projects that the growing use of wearable technology for chronic illness management, preventive care, and remote patient monitoring will propel the global market for wearable healthcare devices to reach $184,403.60 million by 2031. Predictive analytics will be essential to using the volume of data provided by wearables as they become more advanced and commonplace in order to improve patient outcomes and encourage proactive health care.
Advancements in Artificial Intelligence and Machine Learning Algorithms
Predictive analytics, artificial intelligence (AI), and machine learning (ML) technologies together have the potential to completely transform clinical decision-making and healthcare delivery. Predictive models driven by artificial intelligence have never been faster or more accurate in analyzing large, complicated datasets, seeing minute trends, and producing useful insights. Artificial intelligence (AI)-driven predictive analytics opens up new opportunities for precision medicine and tailored healthcare delivery, from identifying illnesses and forecasting treatment outcomes to streamlining care pathways and forecasting patient outcomes.
Advancements in computer vision, natural language processing (NLP), and deep learning algorithms have led to previously unheard-of levels of predictive analytics in the healthcare industry. These days, machine learning algorithms can extract useful information from multimodal data, such as genetic sequences, unstructured clinical notes, and medical images, and help with data-driven decision-making. Predictive analytics will become more and more important as AI technologies develop and advance because they may improve clinical workflows, increase diagnostic accuracy, and optimize resource allocation in many healthcare settings.
Expansion of Predictive Analytics into Public Health Initiatives
Predictive analytics has the potential to significantly impact population well-being and address more general public health issues in addition to clinical care. These models can detect patterns, anticipate disease outbreaks, and guide focused interventions to enhance community health outcomes by evaluating population-level data, socioeconomic determinants of health, and environmental factors. Predictive analytics is a potent tool for advancing public health activities and influencing health policy decisions, from tracking infectious diseases and monitoring vaccine coverage to tackling social inequities and promoting health equity.
It is vital for pandemic preparedness, response, and recovery, as the COVID-19 pandemic has shown. Public health authorities and policymakers can better prepare for pandemics and protect population health by using predictive models to estimate illness spread, evaluate healthcare capacity, and direct vaccination distribution tactics. We may anticipate seeing a wider integration of predictive analytics into emergency response procedures, public health monitoring systems, and health equality programs as governments and healthcare institutions prioritize investments in predictive analytics infrastructure and capabilities.
Collaboration with Genetic and Genomic Data for Precision Medicine
Precision medicine and personalized healthcare could be revolutionized by combining genomic and genetic data with predictive analytics. Predictive models can maximize therapeutic results, anticipate medication reactions, and customize treatment regimens to the genotypes of individual patients by examining genetic variants, biomarkers, and pharmacogenomic data. Predictive analytics enables healthcare professionals to give tailored interventions that optimize effectiveness while minimizing side effects, from cancer genomics and pharmacogenetics to rare illness detection and prevention.
A study that was published in Nature Genetics demonstrates how predictive analytics can be used to forecast the risk of disease and direct therapeutic decision-making by utilizing genetic and genomic data. Researchers made significant progress in illness prediction, biomarker identification, and therapy development by fusing machine learning algorithms with large-scale genetic information. This opened the door for precision medicine techniques that will transform patient care. Predictive analytics will be essential to realizing the full promise of genomic medicine and launching a new age of individualized treatment as genetic testing tools become more widely available and reasonably priced.
Addressing Ethical and Regulatory Challenges for Widespread Adoption
Predictive analytics has the potential to revolutionize healthcare, but widespread implementation depends on resolving intricate ethical and legal issues. To fully utilize predictive analytics ethically, healthcare institutions must navigate a number of ethical conundrums and legal frameworks, from protecting patient privacy and data security to reducing algorithmic biases and fostering openness.
Health Insurance Portability and Accountability Act (HIPAA), General Data Protection Regulation (GDPR) of the European Union, and other legal standards impose strict requirements for data privacy, consent management, and transparency in healthcare analytics. For the purpose of protecting patient rights, reducing risks, and promoting confidence in predictive analytics solutions, healthcare companies need to put strong data governance policies, ethical principles, and accountability systems into place.
Furthermore, improving health equity and minimizing inequities in the provision of healthcare depends critically on resolving algorithmic biases and guaranteeing fairness in predictive models. Healthcare organizations must use strict validation procedures and transparency measures to make sure predictive analytics solutions respect patient diversity and dignity, uphold ethical standards, and include algorithmic auditing, bias detection, and model explainability and interpretability.
Predictive analytics is a rapidly changing field in healthcare, and to fully realize its transformative potential while defending patient rights and advancing equitable access to high-quality care, we must put ethical principles, patient-centered values, and regulatory compliance first. We can use predictive analytics to build a future where healthcare is proactive, individualized, and accessible to all by encouraging stakeholder engagement, funding education and training, and embracing a culture of responsible innovation.
Conclusion
We have reached an exciting new era in healthcare defined by data-driven insights, technological innovation, and patient-centered care as we conclude our exploration of predictive analytics. This journey has revealed the profound impact of predictive analytics on improving patient outcomes, informing clinical decisions, and transforming healthcare delivery, highlighting its vital role in the future of medicine.
Predictive analytics is a potent tool for healthcare professionals to foresee, mitigate, and manage a wide range of healthcare challenges, from early disease identification and individualized therapy to pandemic preparedness and public health surveillance. Precision medicine, preventative care, and population health management can reach new heights with the use of predictive models, machine learning algorithms, and sophisticated analytics approaches.
But much as we welcome the potential of predictive analytics, we must face the dangers it carries, as well as the moral and legal issues that arise when using it. A concerted effort is needed to respect ethical norms, limit risks, and prioritize patient well-being in the responsible adoption of predictive analytics. These include protecting patient privacy, resolving algorithmic biases, and promoting health fairness and transparency.
With wearable technology, artificial intelligence, and genomic medicine leading the way, predictive analytics in healthcare has a bright future ahead of it. We can leverage the revolutionary power of predictive analytics to build a future where healthcare is proactive, individualized, and accessible to all by adopting a culture of innovation, cooperation, and ongoing learning.
We must not waver in our dedication to patient-centered care, evidence-based practice, and ethical integrity as we head into the future of healthcare analytics. By working together, we can harness the potential of predictive analytics to transform the way healthcare is delivered, enhance patient outcomes, and create a more resilient and healthy planet for future generations.
What are your aspirations for the future of predictive analytics in healthcare? How do you envision its impact on patient care, clinical practice, and healthcare systems? Join the conversation and share your insights as we shape the future of healthcare together.
Thank you for joining us on this journey through the realms of AI and cost-effective call center strategies. For more insightful content, stay tuned to our blogs at Odio.
Leave a Reply